Domain‐specific neural networks improve automated bird sound recognition already with small amount of local data
Lauha, P., Somervuo, P., Lehikoinen, P., Geres, L., Richter, T., Seibold, S., & Ovaskainen, O. (2022). Domain‐specific neural networks improve automated bird sound recognition already with small amount of local data. Methods in Ecology and Evolution, 13(12), 2799-2810. https://doi.org/10.1111/2041-210x.14003
Published in
Methods in Ecology and EvolutionAuthors
Date
2022Copyright
© 2022 The Authors. Methods in Ecology and Evolution published by John Wiley & Sons Ltd on behalf of British Ecological Society.
An automatic bird sound recognition system is a useful tool for collecting data of different bird species for ecological analysis. Together with autonomous recording units (ARUs), such a system provides a possibility to collect bird observations on a scale that no human observer could ever match. During the last decades, progress has been made in the field of automatic bird sound recognition, but recognizing bird species from untargeted soundscape recordings remains a challenge.
In this article, we demonstrate the workflow for building a global identification model and adjusting it to perform well on the data of autonomous recorders from a specific region. We show how data augmentation and a combination of global and local data can be used to train a convolutional neural network to classify vocalizations of 101 bird species. We construct a model and train it with a global data set to obtain a base model. The base model is then fine-tuned with local data from Southern Finland in order to adapt it to the sound environment of a specific location and tested with two data sets: one originating from the same Southern Finnish region and another originating from a different region in German Alps.
Our results suggest that fine-tuning with local data significantly improves the network performance. Classification accuracy was improved for test recordings from the same area as the local training data (Southern Finland) but not for recordings from a different region (German Alps). Data augmentation enables training with a limited number of training data and even with few local data samples significant improvement over the base model can be achieved. Our model outperforms the current state-of-the-art tool for automatic bird sound classification.
Using local data to adjust the recognition model for the target domain leads to improvement over general non-tailored solutions. The process introduced in this article can be applied to build a fine-tuned bird sound classification model for a specific environment.
...


Publisher
Wiley-BlackwellISSN Search the Publication Forum
2041-210XKeywords
Publication in research information system
https://converis.jyu.fi/converis/portal/detail/Publication/159209362
Metadata
Show full item recordCollections
Related funder(s)
European CommissionFunding program(s)
ERC European Research Council, H2020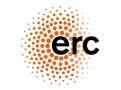

The content of the publication reflects only the author’s view. The funder is not responsible for any use that may be made of the information it contains.
Additional information about funding
Otso Ovaskainen was funded by the Academy of Finland (grant no. 309581), Jane and Aatos Erkko Foundation, Research Council of Norway through its Centres of Excellence Funding Scheme (223257), and the European Research Council (ERC) under the European Union's Horizon 2020 research and in-novation programme (grant agreement No 856506; ERC-synergy project LIFEPLAN).License
Related items
Showing items with similar title or keywords.
-
Hyper-flexible Convolutional Neural Networks based on Generalized Lehmer and Power Means
Terziyan, Vagan; Malyk, Diana; Golovianko, Mariia; Branytskyi, Vladyslav (Elsevier, 2022)Convolutional Neural Network is one of the famous members of the deep learning family of neural network architectures, which is used for many purposes, including image classification. In spite of the wide adoption, such ... -
Causality-Aware Convolutional Neural Networks for Advanced Image Classification and Generation
Terziyan, Vagan; Vitko, Oleksandra (Elsevier, 2023)Smart manufacturing uses emerging deep learning models, and particularly Convolutional Neural Networks (CNNs) and Generative Adversarial Networks (GANs), for different industrial diagnostics tasks, e.g., classification, ... -
Domain-specific transfer learning in the automated scoring of tumor-stroma ratio from histopathological images of colorectal cancer
Petäinen, Liisa; Väyrynen, Juha P.; Ruusuvuori, Pekka; Pölönen, Ilkka; Äyrämö, Sami; Kuopio, Teijo (Public Library of Science (PLoS), 2023)Tumor-stroma ratio (TSR) is a prognostic factor for many types of solid tumors. In this study, we propose a method for automated estimation of TSR from histopathological images of colorectal cancer. The method is based on ... -
Convolutional Neural Network Based Sleep Stage Classification with Class Imbalance
Xu, Qi; Zhou, Dongdong; Wang, Jian; Shen, Jiangrong; Kettunen, Lauri; Cong, Fengyu (IEEE, 2022)Accurate sleep stage classification is vital to assess sleep quality and diagnose sleep disorders. Numerous deep learning based models have been designed for accomplishing this labor automatically. However, the class ... -
Extracting locations from sport and exercise-related social media messages using a neural network-based bilingual toponym recognition model
Liu, Pengyuan; Koivisto, Sonja; Hiippala, Tuomo; van der Lijn, Charlotte; Väisänen, Tuomas; Nurmi, Marisofia; Toivonen, Tuuli; Vehkakoski, Kirsi; Pyykönen, Janne; Virmasalo, Ilkka; Simula, Mikko; Hasanen, Elina; Salmikangas, Anna-Katriina; Muukkonen, Petteri (National Center for Geographic Information and Analysis, 2022)Sport and exercise contribute to health and well-being in cities. While previous research has mainly focused on activities at specific locations such as sport facilities, "informal sport" that occur at arbitrary locations ...