Extreme Minimal Learning Machine
Kärkkäinen, T. (2018). Extreme Minimal Learning Machine. In ESANN 2018 : Proceedings of the 26th European Symposium on Artificial Neural Networks, Computational Intelligence and Machine Learning (pp. 237-242). ESANN. https://www.elen.ucl.ac.be/Proceedings/esann/esannpdf/es2018-72.pdf
Tekijät
Päivämäärä
2018Tekijänoikeudet
© Author, 2018
Extreme Learning Machine (ELM) and Minimal Learning Machine (MLM) are nonlinear and scalable machine learning techniques with randomly generated basis. Both techniques share a step where a matrix of weights for the linear combination of the basis is recovered. In MLM, the kernel in this step corresponds to distance calculations between the training data and a set of reference points, whereas in ELM transformation with a sigmoidal activation function is most commonly used. MLM then needs additional interpolation step to estimate the actual distance-regression based output. A natural combination of these two techniques is proposed here, i.e., to use a distance-based kernel characteristic in MLM in ELM. The experimental results show promising potential of the proposed technique.Extreme Learning Machine (ELM) and Minimal Learning Machine (MLM) are nonlinear and scalable machine learning techniques with randomly generated basis. Both techniques share a step where a matrix of weights for the linear combination of the basis is recovered. In MLM, the kernel in this step corresponds to distance calculations between the training data and a set of reference points, whereas in ELM transformation with a sigmoidal activation function is most commonly used. MLM then needs additional interpolation step to estimate the actual distance-regression based output. A natural combination of these two techniques is proposed here, i.e., to use a distance-based kernel characteristic in MLM in ELM. The experimental results show promising potential of the proposed technique.
...


Julkaisija
ESANNEmojulkaisun ISBN
978-2-87587-047-6Konferenssi
European Symposium on Artificial Neural Networks, Computational Intelligence and Machine LearningKuuluu julkaisuun
ESANN 2018 : Proceedings of the 26th European Symposium on Artificial Neural Networks, Computational Intelligence and Machine Learning
Alkuperäislähde
https://www.elen.ucl.ac.be/Proceedings/esann/esannpdf/es2018-72.pdfJulkaisu tutkimustietojärjestelmässä
https://converis.jyu.fi/converis/portal/detail/Publication/28889038
Metadata
Näytä kaikki kuvailutiedotKokoelmat
Rahoittaja(t)
Suomen AkatemiaRahoitusohjelmat(t)
Akatemiaohjelma, SA; Profilointi, SA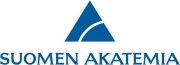
Lisätietoja rahoituksesta
The work of TK has been supported by the Academy of Finland from the projects 311877 (Demo) and 315550 (HNP-AI). The author gratefully acknowledge the role of ESANN in facilitating a collaborative platform with other active research groups of the two methods [11, 12].Lisenssi
Samankaltainen aineisto
Näytetään aineistoja, joilla on samankaltainen nimeke tai asiasanat.
-
Extreme minimal learning machine : Ridge regression with distance-based basis
Kärkkäinen, Tommi (Elsevier BV, 2019)The extreme learning machine (ELM) and the minimal learning machine (MLM) are nonlinear and scalable machine learning techniques with a randomly generated basis. Both techniques start with a step in which a matrix of weights ... -
Problem Transformation Methods with Distance-Based Learning for Multi-Target Regression
Hämäläinen, Joonas; Kärkkäinen, Tommi (ESANN, 2020)Multi-target regression is a special subset of supervised machine learning problems. Problem transformation methods are used in the field to improve the performance of basic methods. The purpose of this article is to test ... -
Artificial intelligence in the cyber security environment
Vähäkainu, Petri; Lehto, Martti (Academic Conferences International, 2019)Artificial Intelligence (AI) is intelligence exhibited by machines. Any system that perceives its environment and takes actions that maximize its chance of success at some goal may be defined as AI. The family of AI ... -
Augmenting machine learning with human insights : the model development for B2B personalization
Yaghtin, Shahrzad; Mero, Joel (Emerald, 2024)Purpose Machine learning (ML) techniques are increasingly important in enabling business-to-business (B2B) companies to offer personalized services to business customers. On the other hand, humans play a critical role in ... -
Taxonomy-Informed Neural Networks for Smart Manufacturing
Terziyan, Vagan; Vitko, Oleksandra (Elsevier, 2024)A neural network (NN) is known to be an efficient and learnable tool supporting decision-making processes particularly in Industry 4.0. The majority of NNs are data-driven and, therefore, depend on training data quantity ...
Ellei toisin mainittu, julkisesti saatavilla olevia JYX-metatietoja (poislukien tiivistelmät) saa vapaasti uudelleenkäyttää CC0-lisenssillä.