Enhancing Identification of Causal Effects by Pruning
Tikka, S., & Karvanen, J. (2018). Enhancing Identification of Causal Effects by Pruning. Journal of Machine Learning Research, 18, 1-23. http://www.jmlr.org/papers/volume18/17-563/17-563.pdf
Published in
Journal of Machine Learning ResearchDate
2018Copyright
© the Authors, 2018.
Causal models communicate our assumptions about causes and e ects in real-world phenomena. Often the interest lies in the identification of the e ect of an action which means deriving an expression from the observed probability distribution for the interventional distribution resulting from the action. In many cases an identifiability algorithm may return a complicated expression that contains variables that are in fact unnecessary. In practice this can lead to additional computational burden and increased bias or ine ciency of estimates when dealing with measurement error or missing data. We present graphical criteria to detect variables which are redundant in identifying causal e ects. We also provide an improved version of a well-known identifiability algorithm that implements these criteria.
Publisher
MIT PressISSN Search the Publication Forum
1532-4435Keywords
Original source
http://www.jmlr.org/papers/volume18/17-563/17-563.pdfPublication in research information system
https://converis.jyu.fi/converis/portal/detail/Publication/28149935
Metadata
Show full item recordCollections
Related funder(s)
Academy of FinlandFunding program(s)
Research profiles, AoF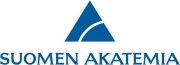
Additional information about funding
We wish to thank Professor Jukka Nyblom for his comments that greatly helped to improve this paper. We also thank the anonymous reviewers for their insightful feedback. The work belongs to the profiling area ”Decision analytics utilizing causal models and multiobjective optimization” (DEMO) supported by Academy of Finland (grant number 311877).Related items
Showing items with similar title or keywords.
-
Clustering and Structural Robustness in Causal Diagrams
Tikka, Santtu; Helske, Jouni; Karvanen, Juha (JMLR, 2023)Graphs are commonly used to represent and visualize causal relations. For a small number of variables, this approach provides a succinct and clear view of the scenario at hand. As the number of variables under study ... -
Simulating Counterfactuals
Karvanen, Juha; Tikka, Santtu; Vihola, Matti (AI Access Foundation, 2024)Counterfactual inference considers a hypothetical intervention in a parallel world that shares some evidence with the factual world. If the evidence specifies a conditional distribution on a manifold, counterfactuals may ... -
Causal Effect Identification from Multiple Incomplete Data Sources : A General Search-Based Approach
Tikka, Santtu; Hyttinen, Antti; Karvanen, Juha (Foundation for Open Access Statistic, 2021)Causal effect identification considers whether an interventional probability distribution can be uniquely determined without parametric assumptions from measured source distributions and structural knowledge on the generating ... -
Causality-Aware Convolutional Neural Networks for Advanced Image Classification and Generation
Terziyan, Vagan; Vitko, Oleksandra (Elsevier, 2023)Smart manufacturing uses emerging deep learning models, and particularly Convolutional Neural Networks (CNNs) and Generative Adversarial Networks (GANs), for different industrial diagnostics tasks, e.g., classification, ... -
Algorithmic issues in computational intelligence optimization : from design to implementation, from implementation to design
Caraffini, Fabio (University of Jyväskylä, 2016)The vertiginous technological growth of the last decades has generated a variety of powerful and complex systems. By embedding within modern hardware devices sophisticated software, they allow the solution of complicated ...