Uniform ergodicity of the iterated conditional SMC and geometric ergodicity of particle Gibbs samplers
Andrieu, C., Lee, A., & Vihola, M. (2018). Uniform ergodicity of the iterated conditional SMC and geometric ergodicity of particle Gibbs samplers. Bernoulli, 24(2), 842-872. https://doi.org/10.3150/15-BEJ785
Julkaistu sarjassa
BernoulliPäivämäärä
2018Tekijänoikeudet
© 2018 ISI/BS. Published by International Statistical Institute; Bernoulli Society for Mathematical Statistics and Probability. Published in this repository with the kind permission of the publisher.
We establish quantitative bounds for rates of convergence and asymptotic variances for iterated conditional sequential Monte Carlo (i-cSMC) Markov chains and associated particle Gibbs samplers [J. R. Stat. Soc. Ser. B. Stat. Methodol. 72 (2010) 269–342]. Our main findings are that the essential boundedness of potential functions associated with the i-cSMC algorithm provide necessary and sufficient conditions for the uniform ergodicity of the i-cSMC Markov chain, as well as quantitative bounds on its (uniformly geometric) rate of convergence. Furthermore, we show that the i-cSMC Markov chain cannot even be geometrically ergodic if this essential boundedness does not hold in many applications of interest. Our sufficiency and quantitative bounds rely on a novel non-asymptotic analysis of the expectation of a standard normalizing constant estimate with respect to a “doubly conditional” SMC algorithm. In addition, our results for i-cSMC imply that the rate of convergence can be improved arbitrarily by increasing N, the number of particles in the algorithm, and that in the presence of mixing assumptions, the rate of convergence can be kept constant by increasing N linearly with the time horizon. We translate the sufficiency of the boundedness condition for i-cSMC into sufficient conditions for the particle Gibbs Markov chain to be geometrically ergodic and quantitative bounds on its geometric rate of convergence, which imply convergence of properties of the particle Gibbs Markov chain to those of its corresponding Gibbs sampler. These results complement recently discovered, and related, conditions for the particle marginal Metropolis–Hastings (PMMH) Markov chain.
...


Julkaisija
International Statistical Institute; Bernoulli Society for Mathematical Statistics and ProbabilityISSN Hae Julkaisufoorumista
1350-7265Asiasanat
Julkaisu tutkimustietojärjestelmässä
https://converis.jyu.fi/converis/portal/detail/Publication/27241862
Metadata
Näytä kaikki kuvailutiedotKokoelmat
Rahoittaja(t)
Suomen AkatemiaRahoitusohjelmat(t)
Akatemiatutkija, SA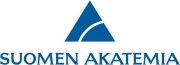
Samankaltainen aineisto
Näytetään aineistoja, joilla on samankaltainen nimeke tai asiasanat.
-
Uniform measure density condition and game regularity for tug-of-war games
Heino, Joonas (International Statistical Institute; Bernoulli Society for Mathematical Statistics and Probability, 2018)We show that a uniform measure density condition implies game regularity for all 2 < p < ∞ in a stochastic game called “tug-of-war with noise”. The proof utilizes suitable choices of strategies combined with estimates for ... -
Coupled conditional backward sampling particle filter
Lee, Anthony; Singh, Sumeetpal S.; Vihola, Matti (Institute of Mathematical Statistics, 2020)The conditional particle filter (CPF) is a promising algorithm for general hidden Markov model smoothing. Empirical evidence suggests that the variant of CPF with backward sampling (CBPF) performs well even with long time ... -
Microscopic Insights Into the Formation of Methanesulfonic Acid–Methylamine–Ammonia Particles Under Acid-Rich Conditions
Liu, Min; Myllys, Nanna; Han, Yaning; Wang, Zhongteng; Chen, Liang; Liu, Wei; Xu, Jing (Frontiers Media SA, 2022)Understanding the microscopic mechanisms of new particle formation under acid-rich conditions is of significance in atmospheric science. Using quantum chemistry calculations, we investigated the microscopic formation ... -
Conditional particle filters with diffuse initial distributions
Karppinen, Santeri; Vihola, Matti (Springer, 2021)Conditional particle filters (CPFs) are powerful smoothing algorithms for general nonlinear/non-Gaussian hidden Markov models. However, CPFs can be inefficient or difficult to apply with diffuse initial distributions, which ... -
Markov chains, MCMC methods and the equi-energy sampler
Tiimonen, Mika (2008)
Ellei toisin mainittu, julkisesti saatavilla olevia JYX-metatietoja (poislukien tiivistelmät) saa vapaasti uudelleenkäyttää CC0-lisenssillä.