Deep learning for flow observables in high energy heavy-ion collisions
Hirvonen, H., Eskola, K. J., & Niemi, H. (2024). Deep learning for flow observables in high energy heavy-ion collisions. In R. Bellwied, F. Geurts, R. Rapp, C. Ratti, A. Timmins, & I. Vitev (Eds.), 30th International Conference on Ultra-Relativistic Nucleus-Nucleus Collisions (Quark Matter 2023) (Article 02002). EDP Sciences. EPJ Web of Conferences, 296. https://doi.org/10.1051/epjconf/202429602002
Julkaistu sarjassa
EPJ Web of ConferencesPäivämäärä
2024Tekijänoikeudet
© 2024 the Authors
We demonstrate how deep convolutional neural networks can be trained to predict 2+1 D hydrodynamic simulation results for flow coefficients, mean-pT and charged particle multiplicity from the initial energy density profile. We show that this method provides results that are accurate enough, so that one can use neural networks to reliably estimate multi-particle flow correlators. Additionally, we train networks that can take any model parameter as an additional input and demonstrate with a few examples that the accuracy remains good. The usage of neural networks can reduce the computation time needed in performing Bayesian analyses with multi-particle flow correlators by many orders of magnitude.
Julkaisija
EDP SciencesEmojulkaisun ISBN
978-2-7598-9126-9Konferenssi
International Conference on Ultra-Relativistic Nucleus-Nucleus CollisionsKuuluu julkaisuun
30th International Conference on Ultra-Relativistic Nucleus-Nucleus Collisions (Quark Matter 2023)ISSN Hae Julkaisufoorumista
2101-6275Julkaisu tutkimustietojärjestelmässä
https://converis.jyu.fi/converis/portal/detail/Publication/220899754
Metadata
Näytä kaikki kuvailutiedotKokoelmat
Rahoittaja(t)
Suomen Akatemia; Euroopan komissioRahoitusohjelmat(t)
Akatemiahanke, SA; Huippuyksikkörahoitus, SA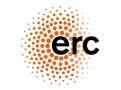

The content of the publication reflects only the author’s view. The funder is not responsible for any use that may be made of the information it contains.
Lisätietoja rahoituksesta
We acknowledge the financial support from the Jenny and Antti Wihuri Foundation, and the Academy of Finland project 330448. This research was funded as a part of the Center of Excellence in Quark Matter of the Academy of Finland (project 346325), the European Research Council project ERC-2018-ADG-835105 YoctoLHC, and the European Union’s Horizon 2020 research and innovation program under grant agreement No 824093 (STRONG-2020). The Finnish IT Center for Science (CSC) is acknowledged for the computing time through the Project jyy2580

Lisenssi
Samankaltainen aineisto
Näytetään aineistoja, joilla on samankaltainen nimeke tai asiasanat.
-
Deep learning for flow observables in ultrarelativistic heavy-ion collisions
Hirvonen, H.; Eskola, K. J.; Niemi, H. (American Physical Society (APS), 2023)We train a deep convolutional neural network to predict hydrodynamic results for flow coefficients, average transverse momenta, and charged particle multiplicities in ultrarelativistic heavy-ion collisions from the initial ... -
Separation of track- and shower-like energy deposits in ProtoDUNE-SP using a convolutional neural network
DUNE Collaboration (Springer Science and Business Media LLC, 2022)Liquid argon time projection chamber detector technology provides high spatial and calorimetric resolutions on the charged particles traversing liquid argon. As a result, the technology has been used in a number of recent ... -
Neuroverkkoihin pohjautuvat koneoppimismallit aivokuvantamismenetelmien tukena
Nordlund, Tuomas (2022)Keinotekoiset neuroverkot ovat tehokkaita laskennallisia työkaluja merkityksellisten piirteiden irroittamiseen suurista datamassoista. Aivokuvantamismenetelmien kehittyessä aivoista saadaan yhä enemmän dataa terveydenhuollon ... -
Tekoälyn hyödyntäminen teollisten valmistusprosessien parantamisessa
Mauno, Topias (2023)Tämän tutkielman tarkoituksena on kuvata tekoälyn nykytilaa ja sen mahdollisia sovelluksia valmistusteollisuudessa. Tekoäly on viime vuosikymmenen aikana noussut merkittäväksi innovaation lähteeksi useilla eri teollisuudenaloilla, ...
Ellei toisin mainittu, julkisesti saatavilla olevia JYX-metatietoja (poislukien tiivistelmät) saa vapaasti uudelleenkäyttää CC0-lisenssillä.