Supplementary data for the article "Machine Learning for Predicting Chemical Potentials of Multifunctional Organic Compounds in Atmospherically Relevant Solutions"
Hyttinen, Noora. Supplementary data for the article "Machine Learning for Predicting Chemical Potentials of Multifunctional Organic Compounds in Atmospherically Relevant Solutions". 10.17011/jyx/dataset/83604
Tekijät
Päivämäärä
2022Tekijänoikeudet
Hyttinen, Noora and University of Jyväskylä
The data set contains the supplementary data of the article "Machine Learning for Predicting Chemical Potentials of Multifunctional Organic Compounds in Atmospherically Relevant Solutions" published in J. Phys. Chem. Lett., https://doi.org/10.1021/acs.jpclett.2c02612. The data includes:
- A machine learning (EMLM) model for predicting chemical potentials of individual conformers of multifunctional organic compounds calculated by the COSMOtherm program
- COSMO-files used for training and testing the EMLM model
- Descriptors and chemical potentials used for the training and testing the model Artikkelin "Machine Learning for Predicting Chemical Potentials of Multifunctional Organic Compounds in Atmospherically Relevant Solutions" lisäaineisto.
Alkuperäislähde
https://nextcloud.jyu.fi/index.php/s/Sa2H5Teycxw9z9Q/downloadAineisto tutkimustietojärjestelmässä
https://converis.jyu.fi/converis/portal/detail/ResearchDataset/155935998
Metadata
Näytä kaikki kuvailutiedotKokoelmat
- Tutkimusdata [250]
Rahoittaja(t)
Academy of Finland; Suomen AkatemiaRahoitusohjelmat(t)
Postdoctoral Researcher, AoF; Tutkijatohtori, SA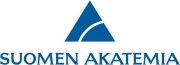
Lisenssi
Samankaltainen aineisto
Näytetään aineistoja, joilla on samankaltainen nimeke tai asiasanat.
-
Machine Learning for Predicting Chemical Potentials of Multifunctional Organic Compounds in Atmospherically Relevant Solutions
Hyttinen, Noora; Pihlajamäki, Antti; Häkkinen, Hannu (American Chemical Society (ACS), 2022)We have trained the Extreme Minimum Learning Machine (EMLM) machine learning model to predict chemical potentials of individual conformers of multifunctional organic compounds containing carbon, hydrogen, and oxygen. The ... -
Supplementary data for the article "The effect of atmospherically relevant aminium salts on water uptake"
Hyttinen, Noora (University of Jyväskylä, 2023)Supplementary data for the article "The effect of atmospherically relevant aminium salts on water uptake". Contains a database of cosmo files used in the COSMOtherm calculations presented in the article. The files are ... -
Supplementary data for the article "Predicting liquid-liquid phase separation in ternary organic-organic-water mixtures"
Hyttinen, Noora (University of Jyväskylä, 2023)Artikkelin "Predicting liquid-liquid phase separation in ternary organic-organic-water mixtures" lisäaineisto. -
The Datafication of Hate : Expectations and Challenges in Automated Hate Speech Monitoring
Laaksonen, Salla-Maaria; Haapoja, Jesse; Kinnunen, Teemu; Nelimarkka, Matti; Pöyhtäri, Reeta (Frontiers Media, 2020)Hate speech has been identified as a pressing problem in society and several automated approaches have been designed to detect and prevent it. This paper reports and reflects upon an action research setting consisting of ... -
A Successful Crowdsourcing Approach for Bird Sound Classification
Lehikoinen, Petteri; Rannisto, Meeri; Camargo, Ulisses; Aintila, Aki; Lauha, Patrik; Piirainen, Esko; Somervuo, Panu; Ovaskainen, Otso (Ubiquity Press, Ltd., 2023)Automated recorders are increasingly used in remote sensing of wildlife, yet automated methods of processing the audio remains challenging. Identifying animal sounds with machine learning provides a solution, but optimizing ...
Ellei toisin mainittu, julkisesti saatavilla olevia JYX-metatietoja (poislukien tiivistelmät) saa vapaasti uudelleenkäyttää CC0-lisenssillä.