Graphical model inference : Sequential Monte Carlo meets deterministic approximations
Lindsten, F., Helske, J., & Vihola, M. (2018). Graphical model inference : Sequential Monte Carlo meets deterministic approximations. In S. Bengio, H. Wallach, H. Larochelle, K. Grauman, N. Cesa-Bianchi, & R. Garnett (Eds.), NeurIPS 2018 : Proceedings of the 32nd Conference on Neural Information Processing Systems. Neural Information Processing Systems Foundation, Inc.. Advances in Neural Information Processing Systems, 31. https://papers.nips.cc/paper/8041-graphical-model-inference-sequential-monte-carlo-meets-deterministic-approximations
Julkaistu sarjassa
Advances in Neural Information Processing SystemsToimittajat
Päivämäärä
2018Tekijänoikeudet
© The Authors & NIPS, 2018.
Approximate inference in probabilistic graphical models (PGMs) can be grouped
into deterministic methods and Monte-Carlo-based methods. The former can often
provide accurate and rapid inferences, but are typically associated with biases
that are hard to quantify. The latter enjoy asymptotic consistency, but can suffer
from high computational costs. In this paper we present a way of bridging the
gap between deterministic and stochastic inference. Specifically, we suggest an
efficient sequential Monte Carlo (SMC) algorithm for PGMs which can leverage
the output from deterministic inference methods. While generally applicable, we
show explicitly how this can be done with loopy belief propagation, expectation
propagation, and Laplace approximations. The resulting algorithm can be viewed as
a post-correction of the biases associated with these methods and, indeed, numerical
results show clear improvements over the baseline deterministic methods as well
as over “plain” SMC.
Julkaisija
Neural Information Processing Systems Foundation, Inc.Konferenssi
Advances in neural information processing systemsKuuluu julkaisuun
NeurIPS 2018 : Proceedings of the 32nd Conference on Neural Information Processing SystemsISSN Hae Julkaisufoorumista
1049-5258
Alkuperäislähde
https://papers.nips.cc/paper/8041-graphical-model-inference-sequential-monte-carlo-meets-deterministic-approximationsJulkaisu tutkimustietojärjestelmässä
https://converis.jyu.fi/converis/portal/detail/Publication/28980157
Metadata
Näytä kaikki kuvailutiedotKokoelmat
Rahoittaja(t)
Suomen AkatemiaRahoitusohjelmat(t)
Akatemiatutkija, SA; Akatemiatutkijan tutkimuskulut, SA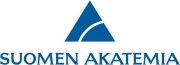
Lisätietoja rahoituksesta
FL has received support from the Swedish Foundation for Strategic Research (SSF) via the project Probabilistic Modeling and Inference for Machine Learning (contract number: ICA16-0015) and from the Swedish Research Council (VR) via the projects Learning of Large-Scale Probabilistic Dynamical Models (contract number: 2016-04278) and NewLEADS – New Directions in Learning Dynamical Systems (contract number: 621-2016-06079). JH and MV have received support from the Academy of Finland (grants 274740, 284513 and 312605).

Lisenssi
Samankaltainen aineisto
Näytetään aineistoja, joilla on samankaltainen nimeke tai asiasanat.
-
Modeling Forest Tree Data Using Sequential Spatial Point Processes
Yazigi, Adil; Penttinen, Antti; Ylitalo, Anna-Kaisa; Maltamo, Matti; Packalen, Petteri; Mehtätalo, Lauri (Springer, 2022)The spatial structure of a forest stand is typically modeled by spatial point process models. Motivated by aerial forest inventories and forest dynamics in general, we propose a sequential spatial approach for modeling ... -
Bayesian Modeling of Sequential Discoveries
Zito, Alessandro; Rigon, Tommaso; Ovaskainen, Otso; Dunson, David B. (Taylor & Francis, 2022)We aim at modelling the appearance of distinct tags in a sequence of labelled objects. Common examples of this type of data include words in a corpus or distinct species in a sample. These sequential discoveries are often ... -
Optimization of Linearized Belief Propagation for Distributed Detection
Abdi, Younes; Ristaniemi, Tapani (IEEE, 2020)In this paper, we investigate distributed inference schemes, over binary-valued Markov random fields, which are realized by the belief propagation (BP) algorithm. We first show that a decision variable obtained by the BP ... -
A Statistical Model of Spine Shape and Material for Population-Oriented Biomechanical Simulation
Sun, Xiaobang; Wang, Hongkai; Wang, Weiying; Li, Nannan; Hämäläinen, Timo; Ristaniemi, Tapani; Liu, Changjian (Institute of Electrical and Electronics Engineers (IEEE), 2021)In population-oriented ergonomics product design and musculoskeletal kinetics analysis, digital spine models of different shape, pose and material property are in great demand. The purpose of this study was to construct a ... -
Clustering ball possession duration according to players’ role in football small-sided games
Coutinho, Diogo; Gonçalves, Bruno; Laakso, Timo; Travassos, Bruno (Public Library of Science (PLoS), 2022)This study aimed to explore which offensive variables best discriminate the ball possession duration according to players specific role (defenders, midfielders, attackers) during a Gk+3vs3+Gk football small-sided games. ...
Ellei toisin mainittu, julkisesti saatavilla olevia JYX-metatietoja (poislukien tiivistelmät) saa vapaasti uudelleenkäyttää CC0-lisenssillä.