Applying machine learning methods for the analysis of two-dimensional mass spectra
Gao, Z., Solders, A., Al-Adili, A., Beliuskina, O., Eronen, T., Kankainen, A., Lantz, M., Moore, I. D., Nesterenko, D. A., Penttilä, H., Pomp, S., Sjöstrand, H., the IGISOL team. (2023). Applying machine learning methods for the analysis of two-dimensional mass spectra. European Physical Journal A, 59, Article 169. https://doi.org/10.1140/epja/s10050-023-01080-x
Julkaistu sarjassa
European Physical Journal APäivämäärä
2023Oppiaine
KiihdytinlaboratorioResurssiviisausyhteisöHyvinvoinnin tutkimuksen yhteisöAccelerator LaboratorySchool of Resource WisdomSchool of WellbeingTekijänoikeudet
© The Author(s) 2023
In a measurement of isomeric yield-ratios in fission, the Phase-Imaging Ion-Cyclotron-Resonance technique, which projects the radial motions of ions in the Penning trap (JYFLTRAP) onto a position-sensitive micro-channel plate detector, has been applied. To obtain the yield ratio, that is the relative population of two states of an isomer pair, a novel analysis procedure has been developed to determine the number of detected ions in each state, as well as corrections for the detector efficiency and decay losses. In order to determine the population of the states in cases where their mass difference is too small to reach full separation, a Bayesian Gaussian Mixture model was implemented. The position-dependent efficiency of the micro-channel plate detector was calibrated by mapping it with 133133Cs++ ions, and a Gaussian Process was trained with the position data to construct an efficiency function that could be used to correct the recorded distributions. The obtained numbers of counts of excited and ground-state ions were used to derive the isomeric yield ratio, taking into account decay losses as well as feeding from precursors.
...


Julkaisija
SpringerISSN Hae Julkaisufoorumista
1434-6001Julkaisu tutkimustietojärjestelmässä
https://converis.jyu.fi/converis/portal/detail/Publication/184081412
Metadata
Näytä kaikki kuvailutiedotKokoelmat
Rahoittaja(t)
Suomen Akatemia; Euroopan komissioRahoitusohjelmat(t)
Akatemiatutkija, SA; EU:n 7. puiteohjelma (FP7)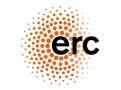

The content of the publication reflects only the author’s view. The funder is not responsible for any use that may be made of the information it contains.
Lisätietoja rahoituksesta
This work was supported by the Swedish research council Vetenskapsrådet (Ref. No. 2017-06481), the European Commission within the Seventh Framework Programme through Fission-2013-CHANDA (Project No. 605203), the Swedish Radiation Safety Authority (SSM). A. Kankainen and D. A. Nesterenko acknowledge the funding from the European Union’s Horizon 2020 research and innovation program under grant agreement No. 771036 (ERC CoG MAIDEN). T. Eronen acknowledges the funding from Academy of Finland (Project No. 295207). We thank Georg Schnabel and Joachim Hansson for fruitful discussions on applying machine learning methods. Open access funding provided by Uppsala University.

Lisenssi
Samankaltainen aineisto
Näytetään aineistoja, joilla on samankaltainen nimeke tai asiasanat.
-
First-forbidden transitions in reactor antineutrino spectra
Hayen, L.; Kostensalo, Joel; Severijns, N.; Suhonen, Jouni (American Physical Society, 2019)We study the dominant forbidden transitions in the antineutrino spectra of the fission actinides from 4 MeV onward using the nuclear shell model. Through explicit calculation of the shape factor, we show the expected changes ... -
Rippling of two-dimensional materials by line defects
Kähärä, Topi; Koskinen, Pekka (American Physical Society (APS), 2020)Two-dimensional materials and their mechanical properties are known to be profoundly affected by rippling deformations. However, although ripples are fairly well understood, less is known about their origin and controlled ... -
Polariton-assisted long-distance energy transfer between excitons in two-dimensional semiconductors
Pajunpää, Tuomas; Nigmatulin, Fedor; Akkanen, Suvi-Tuuli; Fernandez, Henry; Groenhof, Gerrit; Sun, Zhipei (American Physical Society (APS), 2024)Strong exciton-photon coupling offers an effective path for polariton-mediated long-distance coherent energy transfer (ET) between excitonic states. Here, we demonstrate strong coupling between excitons in WS2 monolayers, ... -
Spectral analysis and quantum chaos in two-dimensional nanostructures
Luukko, Perttu (University of Jyväskylä, 2015)This thesis describes a study into the eigenvalues and eigenstates of twodimensional (2D) quantum systems. The research is summarized in four scientific publications by the author. The underlying motivation for this work ... -
Lyapunov quantities and limit cycles in two-dimensional dynamical systems : analytical methods, symbolic computation and visualization
Kuznetsova, Olga (University of Jyväskylä, 2011)
Ellei toisin mainittu, julkisesti saatavilla olevia JYX-metatietoja (poislukien tiivistelmät) saa vapaasti uudelleenkäyttää CC0-lisenssillä.