Increasing stability in the linearized inverse Schrödinger potential problem with power type nonlinearities
Lu, S., Salo, M., & Xu, B. (2022). Increasing stability in the linearized inverse Schrödinger potential problem with power type nonlinearities. Inverse Problems, 38(6), Article 065009. https://doi.org/10.1088/1361-6420/ac637a
Julkaistu sarjassa
Inverse ProblemsPäivämäärä
2022Oppiaine
Inversio-ongelmien huippuyksikköMatematiikkaCentre of Excellence in Inverse ProblemsMathematicsTekijänoikeudet
© 2022 IOP Publishing Ltd.
We consider increasing stability in the inverse Schrödinger potential problem with power type nonlinearities at a large wavenumber. Two linearization approaches, with respect to small boundary data and small potential function, are proposed and their performance on the inverse Schrödinger potential problem is investigated. It can be observed that higher order linearization for small boundary data can provide an increasing stability for an arbitrary power type nonlinearity term if the wavenumber is chosen large. Meanwhile, linearization with respect to the potential function leads to increasing stability for a quadratic nonlinearity term, which highlights the advantage of nonlinearity in solving the inverse Schrödinger potential problem. Noticing that both linearization approaches can be numerically approximated, we provide several reconstruction algorithms for the quadratic and general power type nonlinearity terms, where one of these algorithms is designed based on boundary measurements of multiple wavenumbers. Several numerical examples shed light on the efficiency of our proposed algorithms.
...


Julkaisija
IOP PublishingISSN Hae Julkaisufoorumista
0266-5611Asiasanat
Julkaisu tutkimustietojärjestelmässä
https://converis.jyu.fi/converis/portal/detail/Publication/117587718
Metadata
Näytä kaikki kuvailutiedotKokoelmat
Rahoittaja(t)
Euroopan komissio; Suomen AkatemiaRahoitusohjelmat(t)
Huippuyksikkörahoitus, SA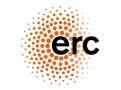

The content of the publication reflects only the author’s view. The funder is not responsible for any use that may be made of the information it contains.
Lisätietoja rahoituksesta
M Salo is supported by the Academy of Finland (Finnish Centre of Excellence in Inverse Modelling and Imaging, Grant 284715) and by the European Research Council under Horizon 2020 (ERC CoG 770924). B Xu is supported by NSFC (No. 12171301 and No. 11801351).Lisenssi
Samankaltainen aineisto
Näytetään aineistoja, joilla on samankaltainen nimeke tai asiasanat.
-
Uniqueness, reconstruction and stability for an inverse problem of a semi-linear wave equation
Lassas, Matti; Liimatainen, Tony; Potenciano-Machado, Leyter; Tyni, Teemu (Elsevier, 2022)We consider the recovery of a potential associated with a semi-linear wave equation on Rn+1, n≥1. We show that an unknown potential a(x,t) of the wave equation □u+aum=0 can be recovered in a Hölder stable way from the map ... -
Optimality of Increasing Stability for an Inverse Boundary Value Problem
Kow, Pu-Zhao; Uhlmann, Gunther; Wang, Jenn-Nan (Society for Industrial & Applied Mathematics (SIAM), 2021)In this work we study the optimality of increasing stability of the inverse boundary value problem (IBVP) for the Schrödinger equation. The rigorous justification of increasing stability for the IBVP for the Schrödinger ... -
The Calderón problem for the fractional Schrödinger equation
Ghosh, Tuhin; Salo, Mikko; Uhlmann, Gunther (Mathematical Sciences Publishers, 2020)We show global uniqueness in an inverse problem for the fractional Schrödinger equation: an unknown potential in a bounded domain is uniquely determined by exterior measurements of solutions. We also show global uniqueness ... -
Inverse problems for elliptic equations with power type nonlinearities
Lassas, Matti; Liimatainen, Tony; Lin, Yi-Hsuan; Salo, Mikko (Elsevier, 2021)We introduce a method for solving Calderón type inverse problems for semilinear equations with power type nonlinearities. The method is based on higher order linearizations, and it allows one to solve inverse problems for ... -
Inverse problems for elliptic equations with fractional power type nonlinearities
Liimatainen, Tony; Lin, Yi-Hsuan; Salo, Mikko; Tyni, Teemu (Elsevier, 2022)We study inverse problems for semilinear elliptic equations with fractional power type nonlinearities. Our arguments are based on the higher order linearization method, which helps us to solve inverse problems for certain ...
Ellei toisin mainittu, julkisesti saatavilla olevia JYX-metatietoja (poislukien tiivistelmät) saa vapaasti uudelleenkäyttää CC0-lisenssillä.