Comparison of cluster validation indices with missing data
Niemelä, M., Äyrämö, S., & Kärkkäinen, T. (2018). Comparison of cluster validation indices with missing data. In ESANN 2018 : Proceedings of the 26th European Symposium on Artificial Neural Networks, Computational Intelligence and Machine Learning (pp. 461-466). ESANN. https://www.elen.ucl.ac.be/Proceedings/esann/esannpdf/es2018-16.pdf
Päivämäärä
2018Tekijänoikeudet
© Authors, 2018
Clustering is an unsupervised machine learning technique, which aims to divide a given set of data into subsets. The number of hidden groups in cluster analysis is not always obvious and, for this purpose, various cluster validation indices have been suggested. Recently some studies reviewing validation indices have been provided, but any experiments against missing data are not yet available. In this paper, performance of ten well-known indices on ten synthetic data sets with various ratios of missing values is measured using squared euclidean and city block distances based clustering. The original indices are modified for a city block distance in a novel way. Experiments illustrate the different degree of stability for the indices with respect to the missing data.
Julkaisija
ESANNEmojulkaisun ISBN
978-2-87587-047-6Konferenssi
European Symposium on Artificial Neural Networks, Computational Intelligence and Machine LearningKuuluu julkaisuun
ESANN 2018 : Proceedings of the 26th European Symposium on Artificial Neural Networks, Computational Intelligence and Machine LearningAsiasanat
Alkuperäislähde
https://www.elen.ucl.ac.be/Proceedings/esann/esannpdf/es2018-16.pdfJulkaisu tutkimustietojärjestelmässä
https://converis.jyu.fi/converis/portal/detail/Publication/28889398
Metadata
Näytä kaikki kuvailutiedotKokoelmat
Rahoittaja(t)
Suomen AkatemiaRahoitusohjelmat(t)
Akatemiaohjelma, SA; Profilointi, SA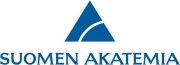
Lisätietoja rahoituksesta
The work has been supported by the Academy of Finland from the project 311737 (DysGeBra). The work has been supported by the Academy of Finland from the projects 311877 (Demo) and 315550 (HNP-AI)Lisenssi
Samankaltainen aineisto
Näytetään aineistoja, joilla on samankaltainen nimeke tai asiasanat.
-
Improving Clustering and Cluster Validation with Missing Data Using Distance Estimation Methods
Niemelä, Marko; Kärkkäinen, Tommi (Springer, 2022)Missing data introduces a challenge in the field of unsupervised learning. In clustering, when the form and the number of clusters are to be determined, one needs to deal with the missing values both in the clustering ... -
Scalable robust clustering method for large and sparse data
Hämäläinen, Joonas; Kärkkäinen, Tommi; Rossi, Tuomo (ESANN, 2018)Datasets for unsupervised clustering can be large and sparse, with significant portion of missing values. We present here a scalable version of a robust clustering method with the available data strategy. Moreprecisely, a ... -
Determination of the Time Window of Event-Related Potential Using Multiple-Set Consensus Clustering
Mahini, Reza; Li, Yansong; Ding, Weiyan; Fu, Rao; Ristaniemi, Tapani; Nandi, Asoke K.; Chen, Guoliang; Cong, Fengyu (Frontiers Media SA, 2020)Clustering is a promising tool for grouping the sequence of similar time-points aimed to identify the attention blocks in spatiotemporal event-related potentials (ERPs) analysis. It is most likely to elicit the appropriate ... -
Clustering ball possession duration according to players’ role in football small-sided games
Coutinho, Diogo; Gonçalves, Bruno; Laakso, Timo; Travassos, Bruno (Public Library of Science (PLoS), 2022)This study aimed to explore which offensive variables best discriminate the ball possession duration according to players specific role (defenders, midfielders, attackers) during a Gk+3vs3+Gk football small-sided games. ... -
Improvements and applications of the elements of prototype-based clustering
Hämäläinen, Joonas (Jyväskylän yliopisto, 2018)
Ellei toisin mainittu, julkisesti saatavilla olevia JYX-metatietoja (poislukien tiivistelmät) saa vapaasti uudelleenkäyttää CC0-lisenssillä.